"More than 15 billion IoT devices will connect to the enterprise infrastructure by 2029.”[1] Finding data is not going to be a challenge, clearly, but taking advantage of it all to drive business outcomes will be.
Combining AI and machine learning (ML) with data collection and processing capabilities of the edge and the cloud may hold the answer. But making the most of that opportunity requires organizations to have application strategies purpose-built to take advantage of all those riches.
While the specific strategies and implementations used to leverage AI will vary between organizations and industries, there is common ground. A recent panel discussion, Strategize for AI from Edge to Cloud, at the 2021 Hitachi Social Innovation Forum (HSIF) unearthed four questions that together provide a framework to shape your strategic thinking. Panelists included Research Director Matt Aslett of 451 Research, Chief Innovation Officer Jason Carolan of Flexentia, CTO for Northern EMEA Tom Christensen of Hitachi Vantara, and Head of Industry Solutions Marketing Lothar Schubert of Hitachi Vantara.
Question 1: How do you develop effective apps in a distributed world?
For 30 years, organizations built secure, walled data centers where they ran monolithic applications. Today that model is out of step. Modern applications thrive by extending their reach to the edge and the cloud while still tapping the data center.
CIOs and their colleagues should adopt an app-first infrastructure mindset to take advantage of the power of the edge and the cloud. The app-first infrastructure prioritizes the breaking down of technical, architectural and social silos to make it simpler to create distributed applications.
This mindset is manifested by insisting that applications developers, operations staff, security people and other technical staff work together to support a distributed environment. Their collaboration will need the support of an app distribution platform such as Kubernetes. Such a platform helps propagate their handiwork from the data center to the edge and the cloud. It also ensures that operators get feedback: visibility they need to understand what is going on “out there.”
The app-first infrastructure is the organizing principle on which AI can be deployed logically and to maximize its impact.
Question 2: What work will happen where?
The philosophical shift to an app-first mindset leads to the question: What goes where? The answer is significant right now. The past year’s social distancing, remote work requirements, and increased need for automation drove massive expansion at the edge. The world is racing along in its adoption of the edge and the deployment of AI to edge processes. Yet many IT leaders are just beginning to make sense of what “edge” even means.
What is the edge? 451 Research’s Matt Aslett shared the following analogy in the HSIF session to help visualize the edge more concretely.
When we look at Saturn with the naked eye or a weak telescope, the “rings” at best appear to be a single, hazy entity. Similarly, a core business based on a traditional data center also seems to be a single, uniform thing. However, when we increase the magnification, the ring of Saturn resolves into first several, then dozens, and even hundreds of discrete rings. As we look closer at an organization, we see greater definition and nuance at its edge in terms of the variety of devices and processing that happens across space.
Aslett postulates that there are two categories of edge:
- The “true edge” refers specifically to devices, intelligent gateways and attached infrastructure.
- The “near edge” is composed of things adjacent to proper edge devices, such as the standalone servers, proximate data centers and network operators.
Keeping this structure in mind helps in visualizing how to optimize the division of labor at the edge. Edge computing aims to move compute processing closer to the devices to overcome network latency and increase the value generated by connected devices. Edge devices will provide much of the data that will train and develop AI models. That topology, therefore, informs where AI resources are located and where AI model output is applied.
Question 3: What connectivity exists today, and what should we anticipate in the near future?
Gateways are a crucial consideration in an app-first infrastructure. They are needed to support north-south communication between the data center, edge and the cloud. Gateways must also enable the east-west communication that occurs laterally between devices and within layers of the stack. Many protocols may be in use, including 3G, 4G, 5G, old LAN networks and proprietary interfaces to legacy systems.
Consider the case of a power grid. The latest just-installed technology at a new transformer station might need to communicate with decades-old distribution boxes to deliver power to a remote farmhouse. It is too costly to put traditional servers or virtual machines at many far-flung locations. Instead, modern, lightweight gateways can store data and support intelligent edge-based software enabled by Kubernetes or other container technology. Those gateways can then not only collect data but also process it and take intelligent action determined by AI.
5G promises to be an important enabler of the edge. With a pace close to wired speeds, 5G allows the creation of wireless networks with high redundancy, greater resiliency and fantastic performance for demanding applications that require low latency and data from multiple sources. Those characteristics will drive decisions that can generate revenue, save money and increase competitiveness through distributed applications.
Question 4: What principles will govern data management in this new landscape?
Organizations already awash in data find that challenge exacerbated by the ever-increasing volume of new data generated by edge devices. Enterprises no longer have the luxury of just choosing to process some data at the edge. Processing data at the edge is necessary to manage the data flow so that it can be stored and further analyzed.
With so much data and the dispersal of processing, it is also critical to consider the need for a single point of truth to maintain control of data and meet compliance requirements. Organizations have become adept at this concerning their structured data, but they are far less certain of their unstructured data. In fact, a Harvard report three years ago found that less than one percent of unstructured data was being used for decision-making.
DataOps is a relatively new term for the agile, automated data management used to enable the app-first infrastructure that features a rich edge component. The term may be new, but there is a high likelihood that your organization has long been thinking along these lines. A joint research project conducted in 2019 by Hitachi Vantara and 451 Research found that 100% of respondents planned to pursue such data management initiatives. As the following chart demonstrates, organizations are increasing their spending across a wide variety of DataOps efforts:
What is most significant about DataOps is that it pairs perfectly with the app-first approach. DataOps enables and connects people, especially nontechnical people, and creates solutions that drive business imperatives.
Answering these four questions today will help an organization move its edge initiatives forward with confidence. AI and ML are becoming easier to implement every day. Organizations should take full advantage of them to drive business success by designing edge and cloud architecture to support a wide variety of AI and ML experimentation projects and solutions.
[1] Smarter with Gartner, Gartner Predicts the Future of Cloud and Edge Infrastructure, Katie Costello, February 8, 2021.
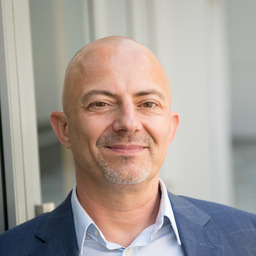
Lothar Schubert
Lothar leads the content marketing and customer advocacy team at Hitachi Vantara. With +15 years of marketing and product management experience, Lothar covers a wide range of topics including digital transformation, analytics, data management and IoT.