In financial services organizations over the past 20 years, we have witnessed traditional process reengineering vastly increasing performance efficiency of operational processes. Organizations have embraced change, driving straight-through processing of transactions to new levels of performance. But their potential to drive further improvements is limited.
The challenge now faced by management is how to drive levels of automation within these organizations, beyond what would normally be considered operating limits.
To achieve this goal, we believe that organizations will need to use highly scalable process-centric technologies. These tools must support both automated and manual processes along with intelligent algorithms, using machine and deep learning methods on top of a distributed consensus-based platform. The intersection between process and these methods will allow organizations to move beyond existing constraints to create core processing platforms that deliver true competitive advantage and market agility.
In starting this journey, it’s important to recognize that automation is driven by data, which is a key enabler and provides the basis for incorporating and leveraging advanced algorithms.
Understanding and codifying core operating and transactional processes and associated data is a foundational component of achieving the highest levels of automation. Consider these areas:
- Automation. This may constitute a normal workflow process or be something that resembles process fragments that are dynamically created based on the type of tasks or computational processes being performed. It is the heart of the automation environment.
- Metrics. Instrumentation of automations is a factor in driving both iterative improvement and enabling an effective operational risk environment. Process execution generates associated metric data.
- Process data. Two types of process data are generated during execution: state data that relates to instance of workflow process being performed and data that is produced and captured and/or written to underlying services.
- Services and applications. Processes may run on top of existing applications or utilize microservices exposed specifically for process automation. Balancing the architectural design of how and where data is persisted with what business logic and transactional scopes is critical to process operation. Consider modern microservices versus legacy application-centric environments.
- Distributed ledger. Process boundaries are not limited by organization, and execution will leverage distributed consensus protocols to ensure both transactional and distributed data integrity. Processes need to be designed from first principles to operate across multiple organizations.
Gaining access and being able to leverage process data will create a foundation for driving efficiencies. These processes will use advanced algorithms that can be deployed across the environment to create automations and increase the improvement of operational efficiencies without significant development.
Intelligent automation methods will drive a significant change across a number of aspects of a financial services organization’s operating model.
They provide the capability to drive very high levels of automation, by leveraging:
- Dynamic composition. Processes can be composed dynamically using intelligent algorithms based on process initiation requests or adapted as part of execution flow, providing a flexible process run-time environment.
- Process operation. Processes will both manage fully automated tasks and assist in the execution of manual tasks by augmenting task performers. This will be enabled by recommendations associated with tasks, autocomplete options based on predictive analytics, and advanced process decisioning, along with routing of tasks and/or optimizing flows paths in real time.
- Advanced controls environment. Operational risk controls are usually based on performing specific maker or checker procedures, or measurements with control breaches only apparent after the fact. Predictive or advanced machine learning algorithms can be applied in real time against control events to provide a forward-looking controls environment.
- Performance. Indicators provide the basis for performance measurement and improvement. These indicators can be used in conjunction with traditional process improvement methodologies and advanced algorithmic analysis applied in real-time or retrospectively. Examples include process improvement simulation and modeling.
Financial services organizations are by default digital. Their products for the most part are dematerialized and can be viewed at a high level as an abstraction of data, process and calculations, or algorithms that are triggered by events over time. Although many financial products are highly automated, it is envisioned that additional automation will not only increase efficiency but also create the opportunity for new innovative products and services.
Change is coming and it’s going to become inevitable for financial services organizations to evolve and adapt their processes and operating models: They will need to take advantage of highly automated and autonomous products and services before their competitors or new market entrants do.
Register for Hitachi Financial Services Summit to Hear More from Thomas De Souza
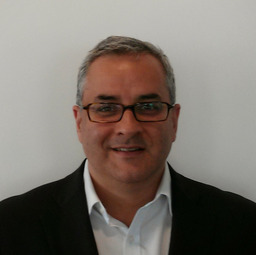
Thomas De Souza
Thomas is a multidisciplinary technologist with an eye for business model innovation and leading-edge data technologies. He has +20 years' experience in financial services with Booz & Co, PwC, a VC-backed FinTech, and global banks including JP Morgan, Deutsche Bank and Citi.